~ 2 min read
Capture and Recognition of Bead Weaving Activities using Hand Skeletal Data and an LSTM Deep Neural Network
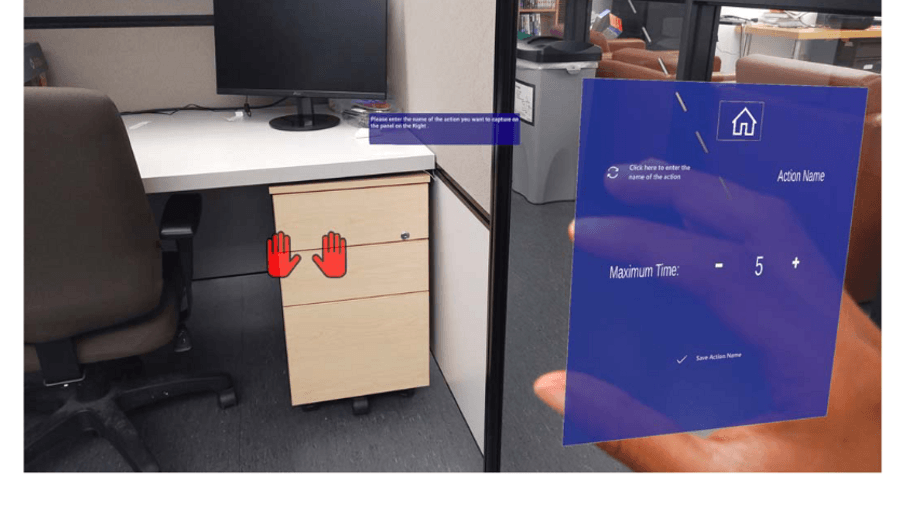
Publication Details
This paper appeared at IEEE AI/VR 2022.
Summary
This short paper presents initial findings related to the research being led by Rowland Goddy-Worlu, a fellow PhD student at the GEM Lab. Rowland’s work involves using Machine Learning for classifying bead-weaving gestures, with the intent of applying that technology to augmented-reality in order to support bead-weaving activities.
My contribution to the work was focused on discussion / consultation with Rowland about his end-to-end ML pipeline, including data pre-processing, model & hyper-parameter selection and evaluation. I appreciate Rowland including me as a co-author on his publication.
Authors
Rowland Goddy-Worlu, Martha Dais Ferreira, Matthew Peachey, James Forren, Claire Nicholas and Derek Reilly
Abstract
Several factors lead to the extinction of tangible cultural heritage, such as globalization, urbanization, intention and accidental neglect, modernization, mechanization, limited usage, migration, and the minimization of skilled practitioners/craft educators and owners. We, the Gesture and Form group, aim to preserve the bead weaving craft, which plays an essential role in culture. We aim to use Augmented reality (AR) and Machine Learning (ML) to preserve this craft. Preservation in this context means passing the technique from one generation to the other, even without a skilled craftsperson. To achieve this, we aim to use an LSTM deep neural network to classify micro activities in the Peyote stitch with the hand skeletal data from the Microsoft Hololens 2. In this paper, we present our preliminary work and a workflow of the ML process involved with a mini project we called “Thumbs-up gesture classification.” Using a single subject participant for the gesture classification, we achieve 100% accuracy on the training and test set, and new data from the device. Finally, we present a future direction for our research.